Is it possible to utilize artificial intelligence to forecast weather in Australia?
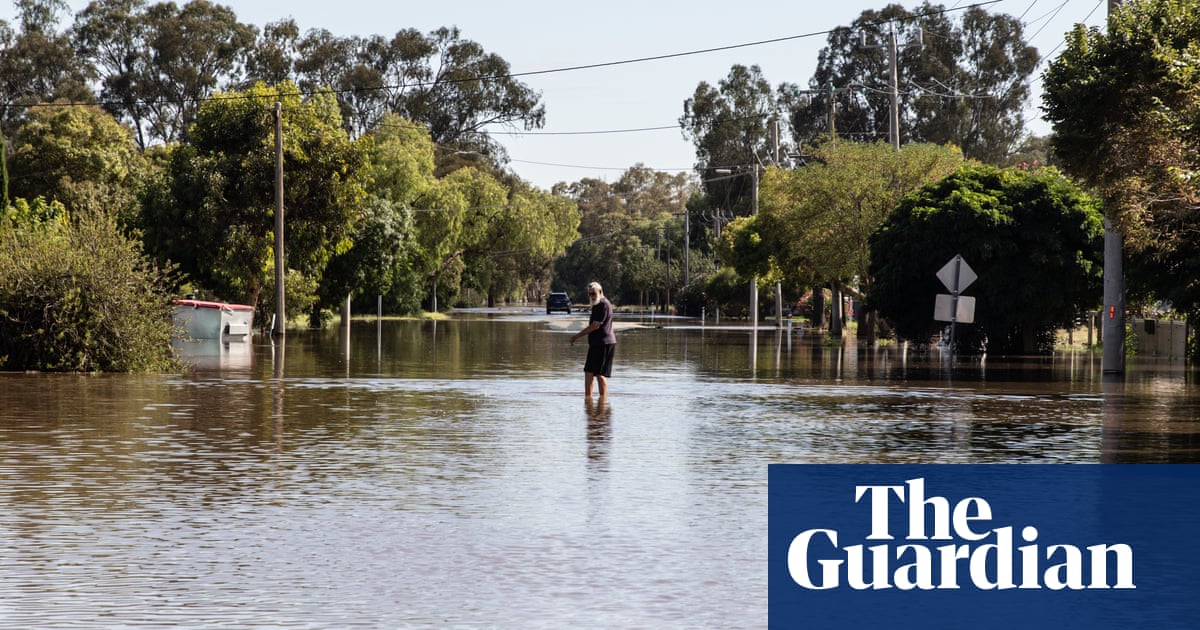
Last year, one evening, Kerry Plowright was relaxing and watching television when his phone alerted him of approaching hail.
“He was amazed upon exiting the door, as there was a deafening noise of hailstones hitting the roofs in Kingscliff, New South Wales,” the man recalls. He quickly protected his cars by placing them under canvas sails, preventing any potential damage.
Many others have also experienced unexpected severe weather during Australia’s ongoing summer of extreme events, just like Plowright. There is a possibility that another tropical cyclone may hit Queensland this season.
The Albanese administration has initiated an investigation into the Bureau of Meteorology and emergency authorities’ issued warnings. This action was taken in response to complaints from councils and other parties, who have raised concerns about the accuracy and timeliness of certain alerts.
However, Plowright’s situation is unique as his warning about hail was prompted by information produced by his company, Early Warning Network.
The Early Warning Network utilizes radar and remote sensors to identify and issue notifications about severe heat, rainfall, and flooding. Its clients include local governments and large insurance companies.
Many private companies have utilized data from BoM or agencies like the ECMWF to provide their services. However, the Early Warning Network is now experimenting with artificial intelligence models that claim to offer a wider range of weather information quickly and affordably.
-
.
Join Guardian Australia’s complimentary morning and afternoon email newsletters to receive a daily summary of news.
According to Plowright, the cost of obtaining [ECMWF] data is exorbitant. However, he believes that a supercomputer is not necessary to produce highly precise forecasts up to 10 days in advance, particularly for severe weather.
He predicts that artificial intelligence will be incredibly effective in predicting weather and eventually climate.
The use of AI to anticipate extreme weather conditions.
Juliette Murphy, a water resources engineer, shares the same enthusiasm. She created FloodMapp in order to provide communities with additional time to prepare for potential floods, after witnessing the devastation caused by floods in Queensland’s Lockyer region in 2011 and later in Calgary, Canada.
FloodMapp utilizes machine learning algorithms in conjunction with traditional hydrology and hydraulic models to analyze large datasets and predict potential flood impacts. According to the company, this process can be completed efficiently even on basic computer systems.
Her clients include Queensland’s fire and emergency services. Its results complement BoM’s, helping authorities decide which homes to evacuate and which roads to close. “That’s important not least because almost half of flood deaths involve people in cars,” Murphy says.
A representative from the Bureau of Meteorology states that the organization has been actively and safely utilizing artificial intelligence technologies for multiple years.
She states that this particular field of study is among the various efforts that the bureau actively engages in to enhance its services for government, emergency management allies, and the general public.
In late 2022, Justin Freeman, a computer scientist, led BoM’s research team in developing machine learning technology before departing to establish his own company, Flowershift.
Flowershift is constructing a geospatial model that utilizes existing observational data. “Our goal is to supplement the current forecast products by filling in missing information,” explains Freeman. This includes offering forecasts for remote areas of Australia and other regions.
Freeman, who continues to do contract work for the bureau, explains that there is now greater flexibility to venture beyond the BoM and utilize cutting-edge technologies. The bureau has also adopted a new set of models that are vastly different from the ones used in the past 50 years.
According to Freeman, models that can efficiently analyze data and provide localized information have numerous potential applications. One example is that farmers could inquire about whether they should spray their crops for the week and receive an explanation for their answer.
“We have only recently gained access to technology like ChatGPT,” he explains. “But in the next two to five years, we can expect even more rapid advancements and improvements.”
The constraints of artificial intelligence.
However, certain researchers in the field of BoM and climate science warn against relying too heavily on AI-based models, such as Google’s GraphCast or Nvidia’s FourCastNet, to enhance numerical models that produce a range of probabilities.
Ignore the promotional newsletter.
after newsletter promotion
One scientist at the bureau believes there is a great potential for using basic methods in weather forecasting and scaling down data from physical models. However, they also caution against relying on these methods for predicting severe weather events.
“We must have a deeper comprehension of phenomena that are significantly different from the usual, especially in regards to climate change.”
Despite the constraints, Sanaa Hobeichi, a researcher at the ARC Centre of Excellence for Climate Extremes, emphasizes that there are still advantages.
Current climate models often have low resolutions, usually around 150km by 150km, which makes it difficult to accurately predict changes in rainfall. For example, a model of this size would encompass the city of Sydney, as well as surrounding mountains and other regions, making it less useful for specific locations.
Google’s GraphCast predictive model has a precision of 28km by 28km, whereas Hobeichi claims that certain AI models can only simulate at a resolution of 5km by 5km.
One difficulty, however, is that machine learning methods may acquire and possibly amplify flaws from the conventional models they are trained on.
Jyoteeshkumar Reddy Papari, a researcher at CSIRO, has observed that the ECMWF was originally doubtful about AI but has now developed its own experimental model. The organization has also featured various other models, such as Google’s, on its website.
According to him, nations without strong meteorological institutions are turning to machine learning models that are readily accessible and simple to understand. As a result, some African countries are utilizing these predictions.
Last year, Google researchers stated that GraphCast performed better than the most accurate operational systems in 90% of 1380 targets. This included predictions for tropical cyclones, atmospheric rivers, and extreme temperatures, surpassing traditional models. Ongoing improvements are being made.
Alvaro Sanchez-Gonzalez, a researcher at Google, frequently references Hurricane Lee as a notable instance where the accuracy of GraphCast’s hurricane trajectory prediction was observed in real-time. This was significant because it diverged from traditional systems and ultimately proved to be the correct trajectory.
“It was identified and confirmed by independent sources in real time.”
The current monitoring of the possible cyclone in the Coral Sea, which is expected to be named Kirrily if it develops by Monday, will also be observed to compare with different models.
Matthew Chantry, the machine learning coordinator at ECMWF, believes that AI models offer an exciting opportunity to complement traditional forecasting methods, while acknowledging that the latter still has its own strengths.
According to him, estimating the intensity of tropical cyclones is a prime example. It remains uncertain if these weaknesses will persist as the technology advances, as it is still in its early stages.
The authorities make decisions using traditional models, which require a powerful supercomputer. However, with the use of AI predictions, this need for computing power is significantly reduced, with some estimates indicating a 1000-fold decrease in energy consumption for forecasting. This could potentially lead to more affordable systems and promote equality.
“We could use the savings to fund bigger groups, providing us with a better understanding of infrequent yet extreme occurrences.”
Regarding forecasting the impacts of a warming planet?
Chantry states that the difficulty of this problem is much greater than that of weather forecasting, as there is a smaller amount of available data. However, in a dynamic climate where there is evidence of a rise in extreme events, accurate prediction of these events would be greatly beneficial.
Source: theguardian.com